The Pitfalls of AI in Scientific Research: Misleading Claims and Reproducibility Issues
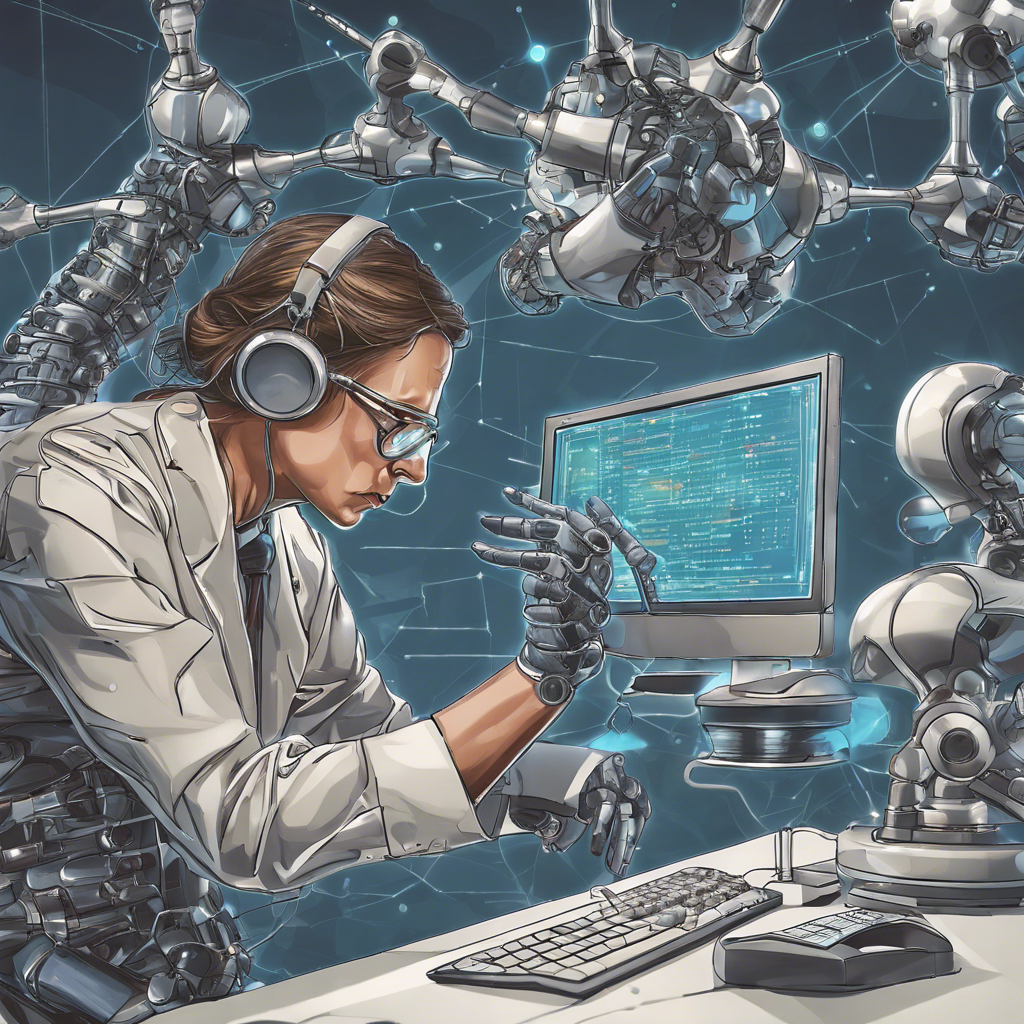
Researchers warn of the widespread problem of misleading claims and reproducibility issues in scientific research due to the ill-informed use of artificial intelligence (AI) and machine learning (ML) techniques.
In recent years, AI and ML have revolutionized scientific research, allowing researchers to uncover patterns in data that are often invisible to the human eye. However, the increasing use of these techniques has also raised concerns about the validity and reliability of the findings. Researchers are now discovering that AI can lead to misleading claims and reproducibility issues, with potentially serious consequences in fields such as biomedicine.
The Problem of Misclassification:
One of the main issues with AI in scientific research is the problem of misclassification. Computer scientists Sanchari Dhar and Lior Shamir discovered that AI algorithms trained on medical images could successfully classify COVID-19 cases even when using blank background sections that showed no body parts at all. This suggests that the AI system was picking up on consistent differences in the backgrounds of the images rather than learning clinically relevant features, rendering it medically useless. Similar cases of misclassification have been found in other areas, from cell types to face recognition.
The Impact on Biomedicine:
Misclassification in biomedicine can have serious consequences, as misdiagnosis can be a matter of life and death. A review of 62 studies using AI to diagnose COVID-19 from chest X-rays or computed tomography scans found that none of the AI models were clinically useful due to methodological flaws or biases in image data sets. These findings highlight the need for rigorous testing and validation of AI models in biomedicine.
The Problem of Data Leakage:
Data leakage is another common issue in AI research. ML algorithms are trained on data sets until they can reliably produce the right outputs for each input. However, if there is insufficient separation between the data used to train the AI system and the data used to test it, data leakage can occur. This can lead to misleading results and an inability to reproduce the findings in real-world settings. It is essential to keep the training set separate from the test set and to run control trials on blank backgrounds to ensure the logical sense of the algorithm’s outputs.
The Need for Reporting Standards and Reproducibility:
To address these issues, researchers have proposed checklists of standards for reporting AI-based science. These checklists include factors such as data quality, details of modeling, and risks of data leakage. However, providing enough details for full reproducibility is challenging, especially in computational sciences like AI. Efforts to improve reproducibility include making methods and data fully open, including source code with submissions, and assessing them against standardized ML reproducibility checklists.
Changing Cultural Norms:
Many researchers believe that changing cultural norms around data presentation and reporting is crucial to addressing the problems with AI in scientific research. This includes providing sufficient information, data, and code to reproduce the results and ensuring transparency in the research process. However, there are challenges, such as privacy concerns and reluctance to release code that is not deemed ready for public scrutiny.
Conclusion:
The ill-informed use of AI in scientific research has led to a proliferation of misleading claims and reproducibility issues. Misclassification and data leakage are among the main problems, particularly in fields like biomedicine. Researchers are calling for reporting standards, reproducibility, and cultural shifts in the research community to address these issues. While some believe that these problems will naturally resolve themselves over time, others emphasize the need for interdisciplinary collaboration and a better understanding of AI’s capabilities and limitations. Ultimately, the goal is to ensure that AI is used responsibly and effectively in scientific research.